Distribution of sum of independent exponentials with random number of summandsRelationship between poisson...
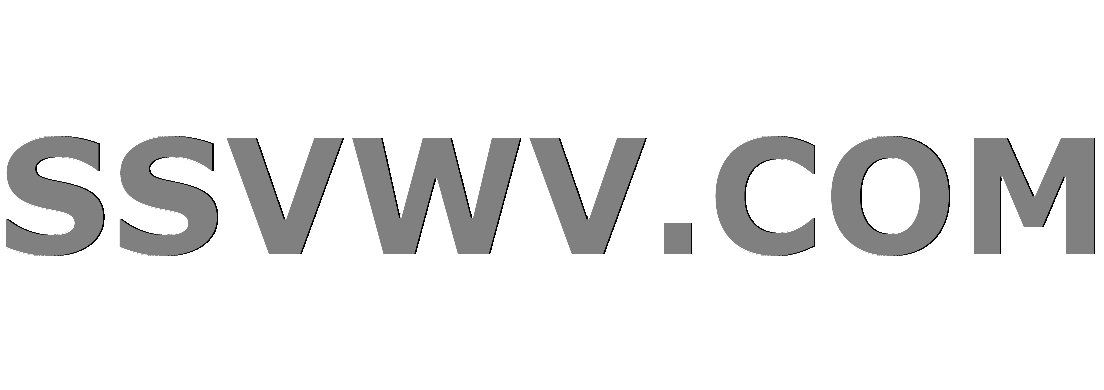
Multi tool use
Why write a book when there's a movie in my head?
When distributing a Linux kernel driver as source code, what's the difference between Proprietary and GPL license?
Coworker is trying to get me to sign his petition to run for office. How to decline politely?
Isn't a semicolon (';') needed after a function declaration in C++?
What is formjacking?
Have any astronauts or cosmonauts died in space?
Why would you use 2 alternate layout buttons instead of 1, when only one can be selected at once
Does the double-bladed scimitar's special attack let you use your ability modifier for the damage of the attack?
What does @ mean in a hostname in DNS configuration?
What does "don't have a baby" imply or mean in this sentence?
Identical projects by students at two different colleges: still plagiarism?
How do I avoid the "chosen hero" feeling?
How can I handle players killing my NPC outside of combat?
How can I make my enemies feel real and make combat more engaging?
Can I legally make a website about boycotting a certain company?
Is layered encryption more secure than long passwords?
Is the tritone (A4 / d5) still banned in Roman Catholic music?
Is the percentage symbol a constant?
Spells that would be effective against a Modern Day army but would NOT destroy a fantasy one
What if you do not believe in the project benefits?
Do the speed limit reductions due to pollution also apply to electric cars in France?
What's the function of the word "ли" in the following contexts?
Multiple null checks in Java 8
What is an explicit bijection in combinatorics?
Distribution of sum of independent exponentials with random number of summands
Relationship between poisson and exponential distributionHow can I efficiently approximate the sum of Bernoulli random variables for any number of summands in partial sum?The weighted sum of two independent Poisson random variablesRandom number from exponential distribution with a scale parameterSum of two independent random variables (application: inter-arrival times)Which parameter should be considered as “scale” parameter for Gamma distribution?Distribution function of an exponential random variableCompound Poisson Distribution with sum of exponential random variablesSum of linear combination of product of exponentials is exponentialDistribution of sum of exponentialsDistribution of a Random Sum
$begingroup$
Let $tau_isimexpleft(lambdaright)$ be independent and identically distributed exponentials with parameter $lambda$. Then, for given $n$, the sum of these values
$$T_n := sum_{i=0}^n tau_i$$
follows an Erlang-Distribution with probability density function
$$pi(T_n=T| n,lambda)={lambda^n T^{n-1} e^{-lambda T} over (n-1)!}quadmbox{for }T, lambda geq 0.$$
I am interested in the distribution of $T_tilde n$ where $tilde n$ is a random variable such that for $tau_a sim exp(lambda_a)$ exponentially distributed, it holds that
$$T_tilde n leq tau_a \T_{tilde{n}+1} > tau_a.$$
In other words, $T_{tilde n}$ is truncated by an exponential distribution. I fail in deriving the distribution of $tilde n$ but maybe there is an easier way:
$$pileft(tilde n = kright) = pileft(T_n < tau_a|n=kright)\
=1-intlimits_{mathbb{R}^+}sumlimits_{n=0}^{k-1}frac{1}{n!}expleft(-(lambda+lambda_a)tau_aright)(taulambda_a)^nlambda_adtau_a.$$
However, just sampling and eye-balling looks to me like this density isn't that ugly:
iter <- 20000
lambda_a <- 1
lambda <- 2
df <- data.frame(tau=rep(NA, iter), a=rep(NA, iter))
for(i in 1:iter){
set.seed(i)
a <- rexp(1, rate = lambda_a)
s <- cumsum(rexp(500, rate = lambda))
df[i,] <- c(max(s[1], s[s<a]), a)
}
library(tidyverse)
ggplot(df %>% gather(), aes(x = value, fill = key)) +
geom_density(alpha = .3) + theme_bw()
distributions exponential-family truncation
$endgroup$
add a comment |
$begingroup$
Let $tau_isimexpleft(lambdaright)$ be independent and identically distributed exponentials with parameter $lambda$. Then, for given $n$, the sum of these values
$$T_n := sum_{i=0}^n tau_i$$
follows an Erlang-Distribution with probability density function
$$pi(T_n=T| n,lambda)={lambda^n T^{n-1} e^{-lambda T} over (n-1)!}quadmbox{for }T, lambda geq 0.$$
I am interested in the distribution of $T_tilde n$ where $tilde n$ is a random variable such that for $tau_a sim exp(lambda_a)$ exponentially distributed, it holds that
$$T_tilde n leq tau_a \T_{tilde{n}+1} > tau_a.$$
In other words, $T_{tilde n}$ is truncated by an exponential distribution. I fail in deriving the distribution of $tilde n$ but maybe there is an easier way:
$$pileft(tilde n = kright) = pileft(T_n < tau_a|n=kright)\
=1-intlimits_{mathbb{R}^+}sumlimits_{n=0}^{k-1}frac{1}{n!}expleft(-(lambda+lambda_a)tau_aright)(taulambda_a)^nlambda_adtau_a.$$
However, just sampling and eye-balling looks to me like this density isn't that ugly:
iter <- 20000
lambda_a <- 1
lambda <- 2
df <- data.frame(tau=rep(NA, iter), a=rep(NA, iter))
for(i in 1:iter){
set.seed(i)
a <- rexp(1, rate = lambda_a)
s <- cumsum(rexp(500, rate = lambda))
df[i,] <- c(max(s[1], s[s<a]), a)
}
library(tidyverse)
ggplot(df %>% gather(), aes(x = value, fill = key)) +
geom_density(alpha = .3) + theme_bw()
distributions exponential-family truncation
$endgroup$
1
$begingroup$
I would recommend not using the same notation for both $tau_i$ and the sum $tau_n$.
$endgroup$
– ukemi
3 hours ago
add a comment |
$begingroup$
Let $tau_isimexpleft(lambdaright)$ be independent and identically distributed exponentials with parameter $lambda$. Then, for given $n$, the sum of these values
$$T_n := sum_{i=0}^n tau_i$$
follows an Erlang-Distribution with probability density function
$$pi(T_n=T| n,lambda)={lambda^n T^{n-1} e^{-lambda T} over (n-1)!}quadmbox{for }T, lambda geq 0.$$
I am interested in the distribution of $T_tilde n$ where $tilde n$ is a random variable such that for $tau_a sim exp(lambda_a)$ exponentially distributed, it holds that
$$T_tilde n leq tau_a \T_{tilde{n}+1} > tau_a.$$
In other words, $T_{tilde n}$ is truncated by an exponential distribution. I fail in deriving the distribution of $tilde n$ but maybe there is an easier way:
$$pileft(tilde n = kright) = pileft(T_n < tau_a|n=kright)\
=1-intlimits_{mathbb{R}^+}sumlimits_{n=0}^{k-1}frac{1}{n!}expleft(-(lambda+lambda_a)tau_aright)(taulambda_a)^nlambda_adtau_a.$$
However, just sampling and eye-balling looks to me like this density isn't that ugly:
iter <- 20000
lambda_a <- 1
lambda <- 2
df <- data.frame(tau=rep(NA, iter), a=rep(NA, iter))
for(i in 1:iter){
set.seed(i)
a <- rexp(1, rate = lambda_a)
s <- cumsum(rexp(500, rate = lambda))
df[i,] <- c(max(s[1], s[s<a]), a)
}
library(tidyverse)
ggplot(df %>% gather(), aes(x = value, fill = key)) +
geom_density(alpha = .3) + theme_bw()
distributions exponential-family truncation
$endgroup$
Let $tau_isimexpleft(lambdaright)$ be independent and identically distributed exponentials with parameter $lambda$. Then, for given $n$, the sum of these values
$$T_n := sum_{i=0}^n tau_i$$
follows an Erlang-Distribution with probability density function
$$pi(T_n=T| n,lambda)={lambda^n T^{n-1} e^{-lambda T} over (n-1)!}quadmbox{for }T, lambda geq 0.$$
I am interested in the distribution of $T_tilde n$ where $tilde n$ is a random variable such that for $tau_a sim exp(lambda_a)$ exponentially distributed, it holds that
$$T_tilde n leq tau_a \T_{tilde{n}+1} > tau_a.$$
In other words, $T_{tilde n}$ is truncated by an exponential distribution. I fail in deriving the distribution of $tilde n$ but maybe there is an easier way:
$$pileft(tilde n = kright) = pileft(T_n < tau_a|n=kright)\
=1-intlimits_{mathbb{R}^+}sumlimits_{n=0}^{k-1}frac{1}{n!}expleft(-(lambda+lambda_a)tau_aright)(taulambda_a)^nlambda_adtau_a.$$
However, just sampling and eye-balling looks to me like this density isn't that ugly:
iter <- 20000
lambda_a <- 1
lambda <- 2
df <- data.frame(tau=rep(NA, iter), a=rep(NA, iter))
for(i in 1:iter){
set.seed(i)
a <- rexp(1, rate = lambda_a)
s <- cumsum(rexp(500, rate = lambda))
df[i,] <- c(max(s[1], s[s<a]), a)
}
library(tidyverse)
ggplot(df %>% gather(), aes(x = value, fill = key)) +
geom_density(alpha = .3) + theme_bw()
distributions exponential-family truncation
distributions exponential-family truncation
edited 3 hours ago
muffin1974
asked 3 hours ago
muffin1974muffin1974
519419
519419
1
$begingroup$
I would recommend not using the same notation for both $tau_i$ and the sum $tau_n$.
$endgroup$
– ukemi
3 hours ago
add a comment |
1
$begingroup$
I would recommend not using the same notation for both $tau_i$ and the sum $tau_n$.
$endgroup$
– ukemi
3 hours ago
1
1
$begingroup$
I would recommend not using the same notation for both $tau_i$ and the sum $tau_n$.
$endgroup$
– ukemi
3 hours ago
$begingroup$
I would recommend not using the same notation for both $tau_i$ and the sum $tau_n$.
$endgroup$
– ukemi
3 hours ago
add a comment |
1 Answer
1
active
oldest
votes
$begingroup$
As detailed in this X validated answer, waiting for a sum of iid exponential $mathcal E(lambda)$ variates to exceed one produces an Poisson $mathcal P(lambda)$ variate $N$. Hence waiting for a sum of iid exponential $mathcal E(lambda)$ variates to exceed $tau_a$ produces an Poisson $mathcal P(tau_alambda)$ variate $N$, conditional on $tau_a$. Therefore
begin{align*}
mathbb P(N=n)&=int_0^infty mathbb P(N=n|tau_a) ,lambda_a e^{-lambda_atau_a},text{d}tau_a\
&= int_0^infty dfrac{(lambdatau_a)^n}{n!},e^{-tau_alambda} ,lambda_a e^{-lambda_atau_a},text{d}tau_a\
&=dfrac{lambda_alambda^n}{n!},int_0^infty tau_a^n,e^{-tau_a(lambda+lambda_a)} ,text{d}tau_a\
&=dfrac{lambda_alambda^n}{n!},dfrac{Gamma(n+1)}{(lambda_a+lambda)^{n+1}}=dfrac{lambda_alambda^n}{(lambda_a+lambda)^{n+1}}
end{align*}
which is a Geometric $mathcal G(lambda_a/{lambda_a+lambda})$ random variable.
Considering now the distribution of$$zeta=sum_{i=1}^N tau_i$$the moment generating function of $zeta$ is
$$mathbb E[e^{zzeta}]=mathbb E[e^{z{tau_1+cdots+tau_N}}]=mathbb E^N[mathbb E^{tau_1}[e^{ztau_1}]^N]=E^N[{lambda/(lambda-z)}^N]=E^N[e^{N(ln lambda-ln (lambda-z))}]$$and the mgf of a Geometric $mathcal G(p)$ variate is
$$varphi_N(z)=dfrac{pe^z}{1-(1-p)e^z}$$Hence the moment generating function of $zeta$ is $$dfrac{pe^{ln lambda-ln (lambda-z)}}{1-(1-(lambda_a/{lambda_a+lambda}))e^{ln lambda-ln (lambda-z)}}=dfrac{p lambda}{ lambda-z-lambda^2/{lambda_a+lambda}}$$
modulo typing mistakes
$endgroup$
$begingroup$
Thanks a lot @Xi'an! Do I get it right that in your notation $p= lambda_a/(lambda_a+lambda)$ ? Because then the moment generating function in the last line is equivalent to $frac{1}{1-zleft(plambdaright)^{-1}}$ which corresponds to the MGF of an exponential distribution..
$endgroup$
– muffin1974
2 hours ago
add a comment |
Your Answer
StackExchange.ifUsing("editor", function () {
return StackExchange.using("mathjaxEditing", function () {
StackExchange.MarkdownEditor.creationCallbacks.add(function (editor, postfix) {
StackExchange.mathjaxEditing.prepareWmdForMathJax(editor, postfix, [["$", "$"], ["\\(","\\)"]]);
});
});
}, "mathjax-editing");
StackExchange.ready(function() {
var channelOptions = {
tags: "".split(" "),
id: "65"
};
initTagRenderer("".split(" "), "".split(" "), channelOptions);
StackExchange.using("externalEditor", function() {
// Have to fire editor after snippets, if snippets enabled
if (StackExchange.settings.snippets.snippetsEnabled) {
StackExchange.using("snippets", function() {
createEditor();
});
}
else {
createEditor();
}
});
function createEditor() {
StackExchange.prepareEditor({
heartbeatType: 'answer',
autoActivateHeartbeat: false,
convertImagesToLinks: false,
noModals: true,
showLowRepImageUploadWarning: true,
reputationToPostImages: null,
bindNavPrevention: true,
postfix: "",
imageUploader: {
brandingHtml: "Powered by u003ca class="icon-imgur-white" href="https://imgur.com/"u003eu003c/au003e",
contentPolicyHtml: "User contributions licensed under u003ca href="https://creativecommons.org/licenses/by-sa/3.0/"u003ecc by-sa 3.0 with attribution requiredu003c/au003e u003ca href="https://stackoverflow.com/legal/content-policy"u003e(content policy)u003c/au003e",
allowUrls: true
},
onDemand: true,
discardSelector: ".discard-answer"
,immediatelyShowMarkdownHelp:true
});
}
});
Sign up or log in
StackExchange.ready(function () {
StackExchange.helpers.onClickDraftSave('#login-link');
});
Sign up using Google
Sign up using Facebook
Sign up using Email and Password
Post as a guest
Required, but never shown
StackExchange.ready(
function () {
StackExchange.openid.initPostLogin('.new-post-login', 'https%3a%2f%2fstats.stackexchange.com%2fquestions%2f393832%2fdistribution-of-sum-of-independent-exponentials-with-random-number-of-summands%23new-answer', 'question_page');
}
);
Post as a guest
Required, but never shown
1 Answer
1
active
oldest
votes
1 Answer
1
active
oldest
votes
active
oldest
votes
active
oldest
votes
$begingroup$
As detailed in this X validated answer, waiting for a sum of iid exponential $mathcal E(lambda)$ variates to exceed one produces an Poisson $mathcal P(lambda)$ variate $N$. Hence waiting for a sum of iid exponential $mathcal E(lambda)$ variates to exceed $tau_a$ produces an Poisson $mathcal P(tau_alambda)$ variate $N$, conditional on $tau_a$. Therefore
begin{align*}
mathbb P(N=n)&=int_0^infty mathbb P(N=n|tau_a) ,lambda_a e^{-lambda_atau_a},text{d}tau_a\
&= int_0^infty dfrac{(lambdatau_a)^n}{n!},e^{-tau_alambda} ,lambda_a e^{-lambda_atau_a},text{d}tau_a\
&=dfrac{lambda_alambda^n}{n!},int_0^infty tau_a^n,e^{-tau_a(lambda+lambda_a)} ,text{d}tau_a\
&=dfrac{lambda_alambda^n}{n!},dfrac{Gamma(n+1)}{(lambda_a+lambda)^{n+1}}=dfrac{lambda_alambda^n}{(lambda_a+lambda)^{n+1}}
end{align*}
which is a Geometric $mathcal G(lambda_a/{lambda_a+lambda})$ random variable.
Considering now the distribution of$$zeta=sum_{i=1}^N tau_i$$the moment generating function of $zeta$ is
$$mathbb E[e^{zzeta}]=mathbb E[e^{z{tau_1+cdots+tau_N}}]=mathbb E^N[mathbb E^{tau_1}[e^{ztau_1}]^N]=E^N[{lambda/(lambda-z)}^N]=E^N[e^{N(ln lambda-ln (lambda-z))}]$$and the mgf of a Geometric $mathcal G(p)$ variate is
$$varphi_N(z)=dfrac{pe^z}{1-(1-p)e^z}$$Hence the moment generating function of $zeta$ is $$dfrac{pe^{ln lambda-ln (lambda-z)}}{1-(1-(lambda_a/{lambda_a+lambda}))e^{ln lambda-ln (lambda-z)}}=dfrac{p lambda}{ lambda-z-lambda^2/{lambda_a+lambda}}$$
modulo typing mistakes
$endgroup$
$begingroup$
Thanks a lot @Xi'an! Do I get it right that in your notation $p= lambda_a/(lambda_a+lambda)$ ? Because then the moment generating function in the last line is equivalent to $frac{1}{1-zleft(plambdaright)^{-1}}$ which corresponds to the MGF of an exponential distribution..
$endgroup$
– muffin1974
2 hours ago
add a comment |
$begingroup$
As detailed in this X validated answer, waiting for a sum of iid exponential $mathcal E(lambda)$ variates to exceed one produces an Poisson $mathcal P(lambda)$ variate $N$. Hence waiting for a sum of iid exponential $mathcal E(lambda)$ variates to exceed $tau_a$ produces an Poisson $mathcal P(tau_alambda)$ variate $N$, conditional on $tau_a$. Therefore
begin{align*}
mathbb P(N=n)&=int_0^infty mathbb P(N=n|tau_a) ,lambda_a e^{-lambda_atau_a},text{d}tau_a\
&= int_0^infty dfrac{(lambdatau_a)^n}{n!},e^{-tau_alambda} ,lambda_a e^{-lambda_atau_a},text{d}tau_a\
&=dfrac{lambda_alambda^n}{n!},int_0^infty tau_a^n,e^{-tau_a(lambda+lambda_a)} ,text{d}tau_a\
&=dfrac{lambda_alambda^n}{n!},dfrac{Gamma(n+1)}{(lambda_a+lambda)^{n+1}}=dfrac{lambda_alambda^n}{(lambda_a+lambda)^{n+1}}
end{align*}
which is a Geometric $mathcal G(lambda_a/{lambda_a+lambda})$ random variable.
Considering now the distribution of$$zeta=sum_{i=1}^N tau_i$$the moment generating function of $zeta$ is
$$mathbb E[e^{zzeta}]=mathbb E[e^{z{tau_1+cdots+tau_N}}]=mathbb E^N[mathbb E^{tau_1}[e^{ztau_1}]^N]=E^N[{lambda/(lambda-z)}^N]=E^N[e^{N(ln lambda-ln (lambda-z))}]$$and the mgf of a Geometric $mathcal G(p)$ variate is
$$varphi_N(z)=dfrac{pe^z}{1-(1-p)e^z}$$Hence the moment generating function of $zeta$ is $$dfrac{pe^{ln lambda-ln (lambda-z)}}{1-(1-(lambda_a/{lambda_a+lambda}))e^{ln lambda-ln (lambda-z)}}=dfrac{p lambda}{ lambda-z-lambda^2/{lambda_a+lambda}}$$
modulo typing mistakes
$endgroup$
$begingroup$
Thanks a lot @Xi'an! Do I get it right that in your notation $p= lambda_a/(lambda_a+lambda)$ ? Because then the moment generating function in the last line is equivalent to $frac{1}{1-zleft(plambdaright)^{-1}}$ which corresponds to the MGF of an exponential distribution..
$endgroup$
– muffin1974
2 hours ago
add a comment |
$begingroup$
As detailed in this X validated answer, waiting for a sum of iid exponential $mathcal E(lambda)$ variates to exceed one produces an Poisson $mathcal P(lambda)$ variate $N$. Hence waiting for a sum of iid exponential $mathcal E(lambda)$ variates to exceed $tau_a$ produces an Poisson $mathcal P(tau_alambda)$ variate $N$, conditional on $tau_a$. Therefore
begin{align*}
mathbb P(N=n)&=int_0^infty mathbb P(N=n|tau_a) ,lambda_a e^{-lambda_atau_a},text{d}tau_a\
&= int_0^infty dfrac{(lambdatau_a)^n}{n!},e^{-tau_alambda} ,lambda_a e^{-lambda_atau_a},text{d}tau_a\
&=dfrac{lambda_alambda^n}{n!},int_0^infty tau_a^n,e^{-tau_a(lambda+lambda_a)} ,text{d}tau_a\
&=dfrac{lambda_alambda^n}{n!},dfrac{Gamma(n+1)}{(lambda_a+lambda)^{n+1}}=dfrac{lambda_alambda^n}{(lambda_a+lambda)^{n+1}}
end{align*}
which is a Geometric $mathcal G(lambda_a/{lambda_a+lambda})$ random variable.
Considering now the distribution of$$zeta=sum_{i=1}^N tau_i$$the moment generating function of $zeta$ is
$$mathbb E[e^{zzeta}]=mathbb E[e^{z{tau_1+cdots+tau_N}}]=mathbb E^N[mathbb E^{tau_1}[e^{ztau_1}]^N]=E^N[{lambda/(lambda-z)}^N]=E^N[e^{N(ln lambda-ln (lambda-z))}]$$and the mgf of a Geometric $mathcal G(p)$ variate is
$$varphi_N(z)=dfrac{pe^z}{1-(1-p)e^z}$$Hence the moment generating function of $zeta$ is $$dfrac{pe^{ln lambda-ln (lambda-z)}}{1-(1-(lambda_a/{lambda_a+lambda}))e^{ln lambda-ln (lambda-z)}}=dfrac{p lambda}{ lambda-z-lambda^2/{lambda_a+lambda}}$$
modulo typing mistakes
$endgroup$
As detailed in this X validated answer, waiting for a sum of iid exponential $mathcal E(lambda)$ variates to exceed one produces an Poisson $mathcal P(lambda)$ variate $N$. Hence waiting for a sum of iid exponential $mathcal E(lambda)$ variates to exceed $tau_a$ produces an Poisson $mathcal P(tau_alambda)$ variate $N$, conditional on $tau_a$. Therefore
begin{align*}
mathbb P(N=n)&=int_0^infty mathbb P(N=n|tau_a) ,lambda_a e^{-lambda_atau_a},text{d}tau_a\
&= int_0^infty dfrac{(lambdatau_a)^n}{n!},e^{-tau_alambda} ,lambda_a e^{-lambda_atau_a},text{d}tau_a\
&=dfrac{lambda_alambda^n}{n!},int_0^infty tau_a^n,e^{-tau_a(lambda+lambda_a)} ,text{d}tau_a\
&=dfrac{lambda_alambda^n}{n!},dfrac{Gamma(n+1)}{(lambda_a+lambda)^{n+1}}=dfrac{lambda_alambda^n}{(lambda_a+lambda)^{n+1}}
end{align*}
which is a Geometric $mathcal G(lambda_a/{lambda_a+lambda})$ random variable.
Considering now the distribution of$$zeta=sum_{i=1}^N tau_i$$the moment generating function of $zeta$ is
$$mathbb E[e^{zzeta}]=mathbb E[e^{z{tau_1+cdots+tau_N}}]=mathbb E^N[mathbb E^{tau_1}[e^{ztau_1}]^N]=E^N[{lambda/(lambda-z)}^N]=E^N[e^{N(ln lambda-ln (lambda-z))}]$$and the mgf of a Geometric $mathcal G(p)$ variate is
$$varphi_N(z)=dfrac{pe^z}{1-(1-p)e^z}$$Hence the moment generating function of $zeta$ is $$dfrac{pe^{ln lambda-ln (lambda-z)}}{1-(1-(lambda_a/{lambda_a+lambda}))e^{ln lambda-ln (lambda-z)}}=dfrac{p lambda}{ lambda-z-lambda^2/{lambda_a+lambda}}$$
modulo typing mistakes
answered 3 hours ago


Xi'anXi'an
57.2k895360
57.2k895360
$begingroup$
Thanks a lot @Xi'an! Do I get it right that in your notation $p= lambda_a/(lambda_a+lambda)$ ? Because then the moment generating function in the last line is equivalent to $frac{1}{1-zleft(plambdaright)^{-1}}$ which corresponds to the MGF of an exponential distribution..
$endgroup$
– muffin1974
2 hours ago
add a comment |
$begingroup$
Thanks a lot @Xi'an! Do I get it right that in your notation $p= lambda_a/(lambda_a+lambda)$ ? Because then the moment generating function in the last line is equivalent to $frac{1}{1-zleft(plambdaright)^{-1}}$ which corresponds to the MGF of an exponential distribution..
$endgroup$
– muffin1974
2 hours ago
$begingroup$
Thanks a lot @Xi'an! Do I get it right that in your notation $p= lambda_a/(lambda_a+lambda)$ ? Because then the moment generating function in the last line is equivalent to $frac{1}{1-zleft(plambdaright)^{-1}}$ which corresponds to the MGF of an exponential distribution..
$endgroup$
– muffin1974
2 hours ago
$begingroup$
Thanks a lot @Xi'an! Do I get it right that in your notation $p= lambda_a/(lambda_a+lambda)$ ? Because then the moment generating function in the last line is equivalent to $frac{1}{1-zleft(plambdaright)^{-1}}$ which corresponds to the MGF of an exponential distribution..
$endgroup$
– muffin1974
2 hours ago
add a comment |
Thanks for contributing an answer to Cross Validated!
- Please be sure to answer the question. Provide details and share your research!
But avoid …
- Asking for help, clarification, or responding to other answers.
- Making statements based on opinion; back them up with references or personal experience.
Use MathJax to format equations. MathJax reference.
To learn more, see our tips on writing great answers.
Sign up or log in
StackExchange.ready(function () {
StackExchange.helpers.onClickDraftSave('#login-link');
});
Sign up using Google
Sign up using Facebook
Sign up using Email and Password
Post as a guest
Required, but never shown
StackExchange.ready(
function () {
StackExchange.openid.initPostLogin('.new-post-login', 'https%3a%2f%2fstats.stackexchange.com%2fquestions%2f393832%2fdistribution-of-sum-of-independent-exponentials-with-random-number-of-summands%23new-answer', 'question_page');
}
);
Post as a guest
Required, but never shown
Sign up or log in
StackExchange.ready(function () {
StackExchange.helpers.onClickDraftSave('#login-link');
});
Sign up using Google
Sign up using Facebook
Sign up using Email and Password
Post as a guest
Required, but never shown
Sign up or log in
StackExchange.ready(function () {
StackExchange.helpers.onClickDraftSave('#login-link');
});
Sign up using Google
Sign up using Facebook
Sign up using Email and Password
Post as a guest
Required, but never shown
Sign up or log in
StackExchange.ready(function () {
StackExchange.helpers.onClickDraftSave('#login-link');
});
Sign up using Google
Sign up using Facebook
Sign up using Email and Password
Sign up using Google
Sign up using Facebook
Sign up using Email and Password
Post as a guest
Required, but never shown
Required, but never shown
Required, but never shown
Required, but never shown
Required, but never shown
Required, but never shown
Required, but never shown
Required, but never shown
Required, but never shown
E9,FOIp V,csGnMPLphz
1
$begingroup$
I would recommend not using the same notation for both $tau_i$ and the sum $tau_n$.
$endgroup$
– ukemi
3 hours ago